You could presume Tesla to be a well-known name in the automobile industry when you think of them. Tesla, a pioneer in electric automobiles, is without a doubt. However, they are a technological firm, which is the secret to their success.
One of the things that have made their business successful is the use of artificial intelligence technologies. The full automation of Tesla’s vehicles is one of the company’s current top priorities, and to achieve this aim, they are utilizing AI and its many components.
By announcing its arrival at the beginning of 2021, Tesla created a stir on the subcontinent. Elon Musk is almost ready to establish Bangalore, India, as Tesla India’s manufacturing hub.
AI experts in India cheered as the memes and tweets regarding how the much-praised “Self-driving Cars” will operate in India continued.
An entire wave of artificial intelligence that will eventually rule the globe is just getting started.
This post will examine in depth how Tesla is integrating AI into its system, including specifics and other information.
So, how does AI Teaches Autonomous Driving in Cars?
Autonomous vehicles continually analyze data from their sensors and machine vision cameras in order to be able to drive independently. They then utilize this data to decide what to do next.
They employ AI to comprehend and predict the next moves of bicycles, pedestrians, and autos. They can use this information to quickly plan their actions and make split-second decisions.
Should the automobile continue in its present lane or should it switch lanes? Should it continue where it is or pass the automobile in front of them? When should the vehicle decelerate or speed up?
Tesla has to gather the appropriate data to train the algorithms and feed its AIs in order to make cars completely autonomous. Better performance will always result from more training data, and Tesla shines in this area.
The fact that Tesla crowdsources all of its data from the hundreds of thousands of Tesla vehicles that are now on the road gives them a competitive advantage. Both internal and exterior sensors track how Teslas behave in a variety of circumstances.
They also gather information on driver behavior, including how they respond to certain circumstances and how frequently they touch the steering wheel or dashboard.
“Imitation learning” is the name of Tesla’s strategy. Millions of real drivers throughout the world make judgments, respond, and move, and their algorithms learn from those actions. All those kilometers result in incredibly sophisticated autonomous vehicles.
Their tracking system is really advanced. For instance, Tesla stores a data snapshot of the moment, adds it to the data set and then recreates an abstract representation of the world using color-coded shapes that the neural network can learn from. This happens when a Tesla vehicle predicts the behavior of a car or bicycle incorrectly.
Other businesses developing autonomous vehicles rely on synthetic data, which is significantly less effective than the real-world data used by Tesla to train its AIs (for instance, driving behavior from video games like Grand Theft Auto).
We will now examine Tesla components that take advantage of AI.
Tesla components that take advantage of AI
Camera & Sensors
The responsibilities that Tesla must complete are pretty well-known. All of these operations, from lane identification to pedestrian tracking, are carried out in real-time. Tesla operated with the help of 8 cameras for this reason. Additionally, the presence of this many cameras assures that there is no blind zone and that the whole area around the car is covered.
It’s true what you just read! no LIDAR No system for high-definition mapping. Tesla wants to use just computer vision, machine learning, and camera video feeds to create the auto-pilot model. Convolutional Neural Networks (CNNs) are then used to analyze the raw video in order to track and detect objects.
Tesla autopilot also has radar and ultrasonic sensors in addition to cameras. The radar is used to detect and measure the separation between vehicles and other objects. In order to optimize driver safety, the ultrasonic sensors also function in accordance with monitoring closeness with passive objects.
In order to understand the surroundings of the car and make the autopilot capabilities as responsive as possible, neural networks are integrated with the Tesla hardware.
Tesla FSD Chip -3
For improved performance and safety on the roads, Tesla systems include two AI processors. The Tesla system strives to be error-free. Even if one unit fails, the automobile can still function using the extra units because of the backup power and data input sources.
Tesla uses these extra measures to make sure the cars are well-equipped to avoid collisions in the event of an unforeseen failure. Only the human brain can execute more operations per second than the new Tesla microprocessor (1 quadrillion operations per sec). That is about 21 times more potent than the Tesla Nvidia microchips that were previously in use.
Tesla is undoubtedly a market leader for fully autonomous locomotives, but it is still a long way from producing a cutting-edge autopilot car.
In the future, an automobile with the qualities we outlined in this essay will undoubtedly become commonplace. Tesla has created its own cutting-edge AI processors and neural network architecture.
Neural Network Training
The model must also be trained after the neural networks have been created. We are aware that Tesla has put in place a wide range of libraries and tools in order to allow cutting-edge computer vision capabilities.
Pytorch, which was created by Facebook’s AI Research department, is one such framework (FAIR). PyTorch is used by the Tesla tech stack to train the deep learning model.
It’s noteworthy that Tesla doesn’t rely on maps or LIDAR to achieve complete autonomy. The cameras and pure computer vision are used exclusively, and everything is done in real-time.
Tesla employs Pytorch for training as well as various auxiliary activities like automated workflow scheduling, calibration of model thresholds, thorough assessment, passive testing, simulation tests, etc.
Tesla spends roughly 70,000 GPU hours training 48 networks that make 1,000 distinct predictions. This training is ongoing, not just once. We are aware that artificial intelligence is an iterative process that advances over time. As a result, all 1000 separate forecasts remain accurate and never falter.
HydraNet
There are around 100 jobs under progress at any given time, even when a car is not moving and is most likely at a crossroads. Using a neural network for every task is costly and ineffective. Massive amounts of information are processed in real-time by the AI in Tesla vehicles.
As a result, the ResNet-50 shared backbone, which can process 1000 x 1000 pictures at once, serves as the central processing unit for the Computer Vision workflow.
Near the top of the network, the HydraNet neural network design divides into several branches (or heads). By having each micro-batch of training data be weighted differently for the many heads, these heads are taught independently and learn distinct things.
Of course, there are several instances of these HydraNets working together to process the AI for the vehicles. Each HydraNet’s information is utilized to remedy recurring problems.
For instance, a task can be active to handle stop signs, another to deal with pedestrians, and yet another to examine traffic signals. These distinct duties are all operated by a common backbone.
According to the HydraNet architecture, just a small fraction of the enormous neural network is needed for each of these tasks.
This is quite similar to transfer learning, where distinct blocks are trained for a common block for certain related tasks. The backbones of HydraNets are trained on a variety of things, whereas the heads are taught on particular jobs.
This decreases the amount of time needed to train the model and speeds up inference.
Tesla Autopilot
Cars with autopilot capabilities can autonomously steer, accelerate, and halt in a lane. It is constructed using deep neural network concepts. It observes the area surrounding the car using cameras, ultrasonic sensors, and radar.
The drivers are made aware of their surroundings by the sensors and cameras, and this information is analyzed in a matter of milliseconds to assist make driving safer and less stressful.
In bright, dark, and various weather circumstances, radar is utilized to observe and estimate the space surrounding automobiles. In every situation, ultraviolet methods determine closeness, and passive video identifies objects nearby and promotes safe driving.
Additionally, autopilot is designed to aid the driver and does not transform a Tesla into a self-driving vehicle. It is common practice to warn drivers to keep their hands on the wheel.
A series of alerts to take the wheel are triggered if you don’t. If ignored much longer, the automobile begins to slow down before coming to a halt. By braking, turning, or deactivating the cruise control stalk, drivers can always override autopilot functions.
Bird’s Eye View
The pictures that Tesla hardware interprets often could need extra dimensions. The Bird’s Eye View feature makes it easier to gauge farther distances and offers a more accurate representation of the outside world.
It is a visual monitoring system that “renders” a top view image of a car to make parking simple and navigating small places easier. Without having to provide a lame justification about your parking abilities, you can now safely take the wheel.
Future of Tesla
If you’re searching for a mid-size SUV with a strong range, the 2022 Tesla Model Y is a fantastic starting point for EVs. Due to regular software upgrades, the Model Y is constantly changing, much like many of Tesla’s other products.
By enhancing safety and functionality, these upgrades help your automobile be more useful. For people who need to travel long distances with family and various luggage, the roomy body and access to Tesla’s Supercharger network make it a wonderful choice.
Since its start, Tesla has benefited from data from its current customer base, and its work on autonomous vehicles is a part of its ongoing ambition to place AI at the core of all its operations.
AI and big data will continue to be Elon Musk and his team at Tesla’s faithful allies as they move into their newest initiatives including their aspirations to transform the electric grid with their home solar power panels.
Conclusion
Tesla, a company that is recognized as one of the market’s most aggressive innovators, has always made data collecting and analysis its most powerful tool. They followed the same rules when it came to creating their own chips.
The business has developed autonomous vehicles that have the potential to completely change how we drive automobiles thanks to artificial intelligence and data analysis.
Let’s see how well the platform upholds its promises and develops its business. Where the company will go in the market for autonomous vehicles in the future remains to be seen after harnessing these technologies.
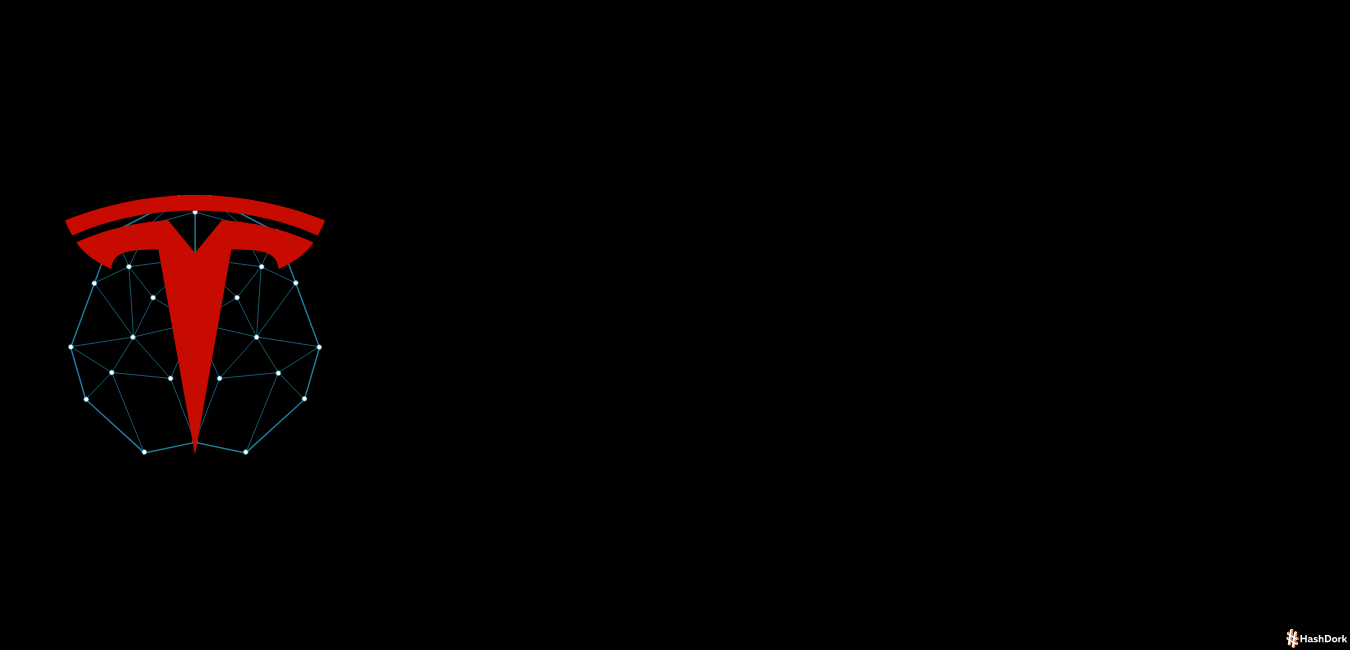
Leave a Reply