With its astonishing qualities and boundless potential for technological improvements, graphene, a single sheet of carbon atoms organized in a two-dimensional lattice, has captured the attention of researchers and scientists all over the world.
The incorporation of machine learning has emerged as a potent technique for opening up new horizons in graphene research and development as the pursuit of harnessing graphene’s transformational qualities grows.
The development of graphene in a variety of industries can be greatly accelerated by machine learning algorithms, which provide special insights, forecasting skills, and optimization chances.
In this article, we will examine how machine learning might transform graphene research by opening up previously unimaginable possibilities for material design, device optimization, and ground-breaking applications that can shortly reshape a variety of sectors.
Graphene: An Atomic-Scale Miracle
Graphene, which is formed from graphite, is a flat, single-layer hexagonal lattice of carbon atoms.
Its atomic-scale thickness and exceptional mechanical, electrical, and thermal qualities have catapulted it into the forefront of scientific inquiry.
With a tensile strength of 200 times that of steel, outstanding electrical conductivity, and incredible heat resistance, graphene is a true wonder material with limitless application potential.
From Electronics to Biomedicine: Incredible Possibilities
Graphene’s extraordinary qualities have aroused considerable excitement in the scientific community, owing principally to its potential to change a variety of industries.
In the field of electronics, graphene’s exceptional electrical conductivity and flexibility open up previously unimagined possibilities for the development of ultra-fast transistors, flexible displays, and high-performance batteries.
Its high thermal conductivity bodes well for efficient heat dissipation in electronic devices.
Furthermore, graphene’s applications go beyond electronics. Graphene composites have demonstrated amazing strength and lightweight properties in the realm of materials science, making them perfect for aircraft applications, construction materials, and even sports equipment.
Graphene’s biocompatibility and impermeability have also accelerated progress in biomedical applications ranging from medication delivery systems to tissue engineering and biosensors.
The Importance of Graphene in Scientific Advancement
The significance of graphene arises from its ability to push the boundaries of scientific knowledge and generate game-changing breakthroughs.
Its discovery has led to new directions in fundamental physics, materials science, and nanotechnology research.
Furthermore, advances in manufacturing techniques have resulted from the synthesis and manipulation of graphene, allowing the research of other two-dimensional materials with similar properties and prospective applications.
Collaborations between scientists, engineers, and industry specialists are becoming increasingly important as researchers continue to uncover graphene’s mysteries.
Graphene is set to affect the future across a wide range of sectors, transforming industries and improving innumerable aspects of our lives, thanks to a rising body of research and technological developments.
So, where does machine learning come to place? Can it help us in this exciting field?
Uncovering the Graphene Landscape with Machine Learning
A potent tool in the study of graphene has turned out to be machine learning. It functions at the intersection of data analysis, computer modeling, and pattern recognition.
So, researchers can gain insightful information and quicken the development of this ground-breaking substance.
Machine learning algorithms can find hidden patterns, anticipate attributes, and enhance graphene-based systems in previously unachievable ways by utilizing enormous amounts of data and computer power.
Unravel the Mysteries of Graphene Using Methods and Models
Machine learning methods cover a wide range of strategies that help in comprehending and working with this unusual substance in the context of graphene research.
Using machine learning algorithms to assess experimental data and determine important characteristics, such as the number of layers, defect density, or thermal conductivity, is one well-known method.
These models can quickly and reliably characterize and manage the quality of graphene materials by processing massive datasets and extracting useful information.
Predictive Modeling: Unlocking the Potential of Graphene
Machine learning models are very useful for forecasting the characteristics and actions of systems based on graphene.
Researchers can develop prediction tools that quicken the design and optimization of graphene-based devices by training models on current data.
For example, machine learning techniques can help with the design of high-performance graphene composites suited for certain applications or with the development of innovative graphene transistors with desired electrical properties.
Researchers can efficiently explore large design areas thanks to machine learning’s predictive capability and find new research directions.
Increasing Discoveries Through Machine Learning-Assisted Research
Machine learning speeds up graphene research by giving scientists effective tools for analyzing complex data, spotting trends, and guiding experimental and theoretical inquiries.
Machine learning algorithms can find unique patterns or connections that would otherwise go unreported because of their ability to process and analyze enormous datasets.
Researchers can use this power to optimize experimental conditions, forecast material behavior, and gain new insights into graphene’s characteristics and possible applications.
Machine Learning Unleashes Accurate Predictions for Graphene Properties
Graphene has been intensively explored due to its extraordinary qualities, however, existing computations frequently produce contradictory conclusions.
Traditional empirical potentials have difficulties in expressing the special properties of graphene.
Machine learning (ML) approaches, on the other hand, offer a promising alternative. The Gaussian Approximation Potential (GAP) approach was used in this study to generate an ML interatomic potential for graphene.
The GAP potential produced accurate predictions of atomic forces, thermal expansion, and phonon spectra after being trained using a large dataset from density functional theory (DFT) simulations, which included energies, forces, and virial stresses.
ML potentials offer a cost-effective and precise option for studying graphene’s dynamic behavior as well as phenomena such as diffusion processes and nuclear quantum effects.
Machine Learning and Graphene Innovations in the Future
Machine learning integration in graphene research provides enormous promise for future developments.
Machine learning techniques are likely to improve our understanding of graphene’s underlying properties, enable the discovery of novel graphene-based materials, and accelerate the development of practical applications as they evolve.
Furthermore, machine learning can play an important role in optimizing graphene manufacturing processes, solving scaling and quality control issues, and therefore opening the road for widespread commercialization and integration of graphene-based technology.
Conclusion
Finally, machine learning has emerged as a game changer in graphene research.
Machine learning enables researchers to expedite discoveries, unravel graphene’s secrets, and drive innovation in this extraordinary material by exploiting its data analytic capabilities, predictive modeling prowess, and optimization potential.
We can expect significant advancements that will change businesses and uncover the full potential of graphene in a variety of disciplines as the synergy between machine learning and graphene research continues to expand.
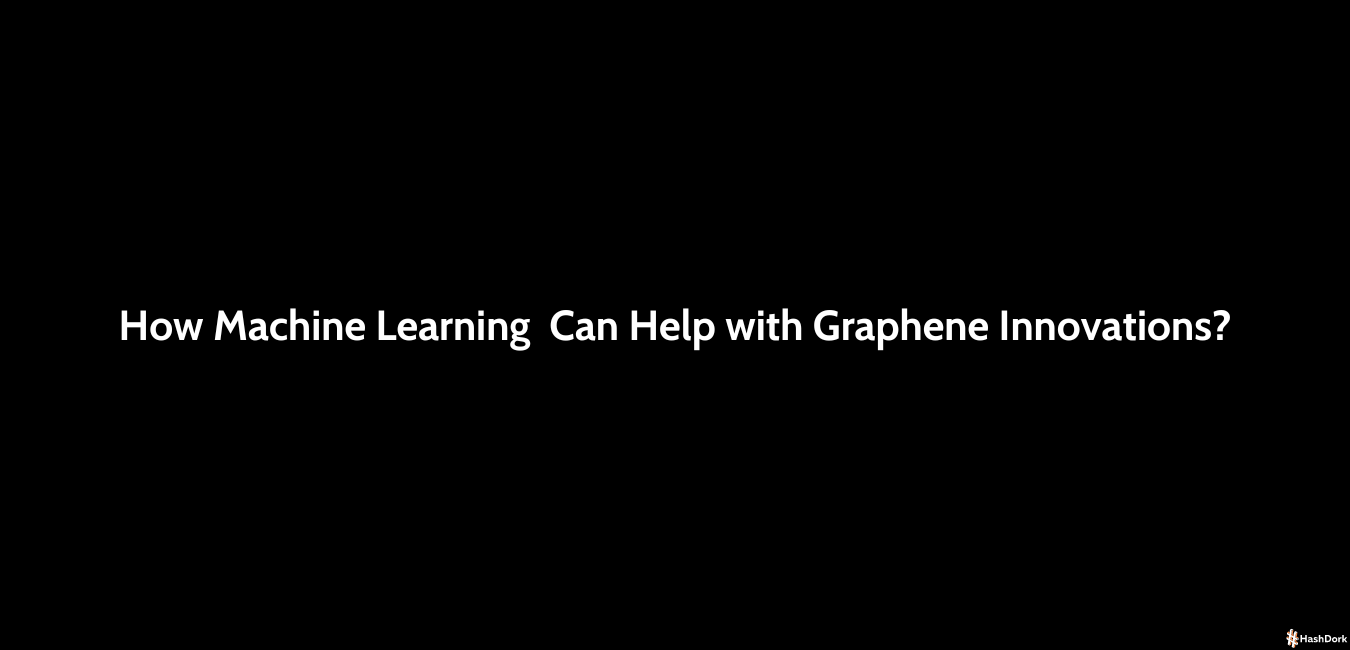
Leave a Reply